The Dashboard
The Netherlands data
Due to the difficulty in comparing the data between the 2 countries, the dashboard was first populated with the Dutch dataset. This provides a good indication of what can be achieved from a consistent, nationwide data collection.
The dashboard contains 5 pages, namely:
-
By municipality
-
By region
-
KPI correlation between parameters
-
KPI growth rate
-
KWR detail
The interactive content below might not be fully accessible.
By municipality
KWR WWTP measurements are compared to nationwide WWTP, infections and hospitalisations on the left side, whereas nationwide WWTP is compared to infections and hospitalisations on the right side. Generally, KWR shows a better match for Utrecht and Amsterdam than the nationwide WWTPs.
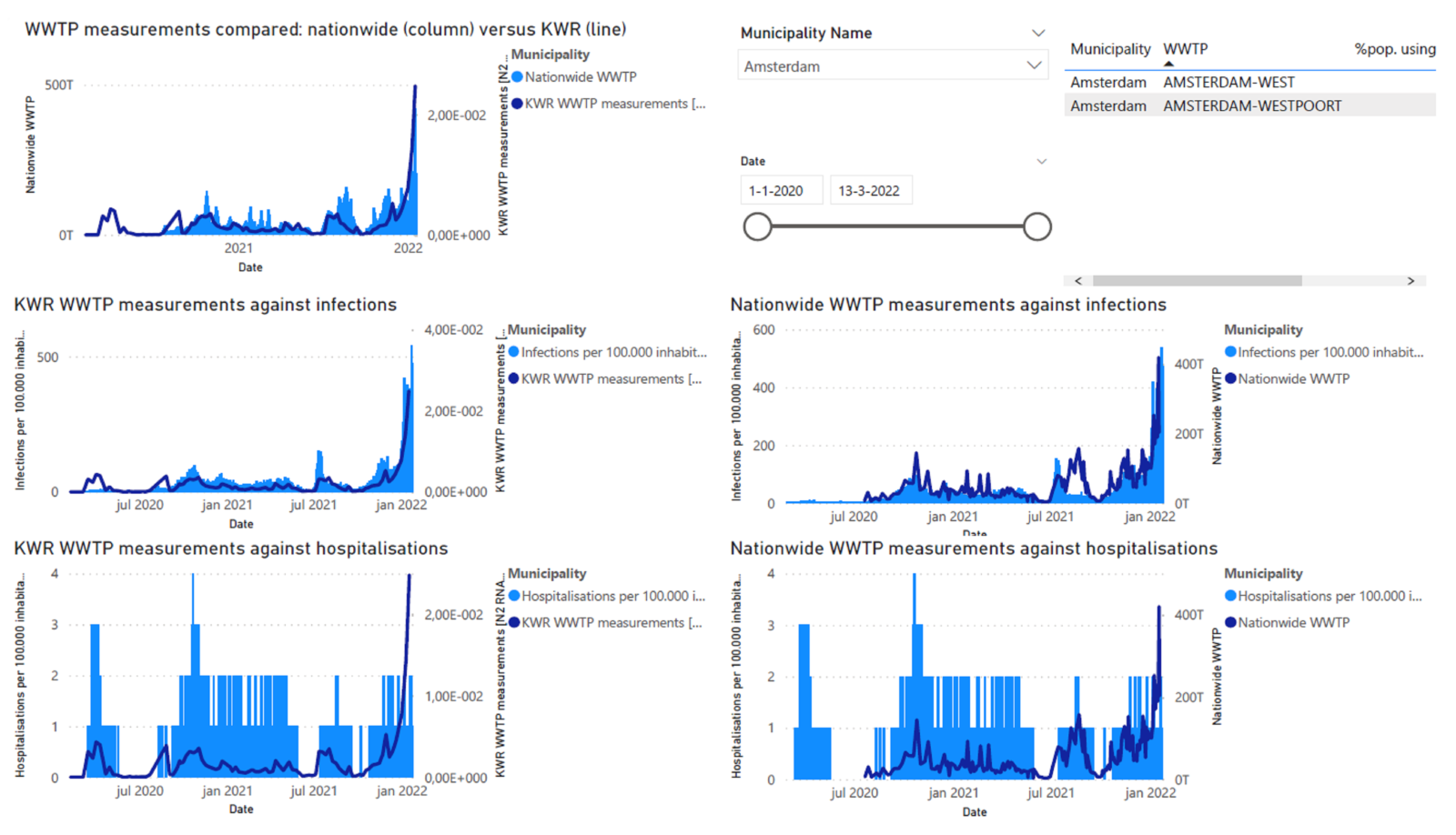
By region
The “by region” page allows for regional selection of multiple municipalities on the top left with ArcGIS selection tools. Next, nationwide WWTP measurements and infections of selected municipalities are shown in the same graph to allow intercomparison. On the right, an additional map shows nationwide WWTP measurements on their sample site. ArcGIS can play progress in time of nationwide WWTP measurements and allows for selection of the exact date and time interval.
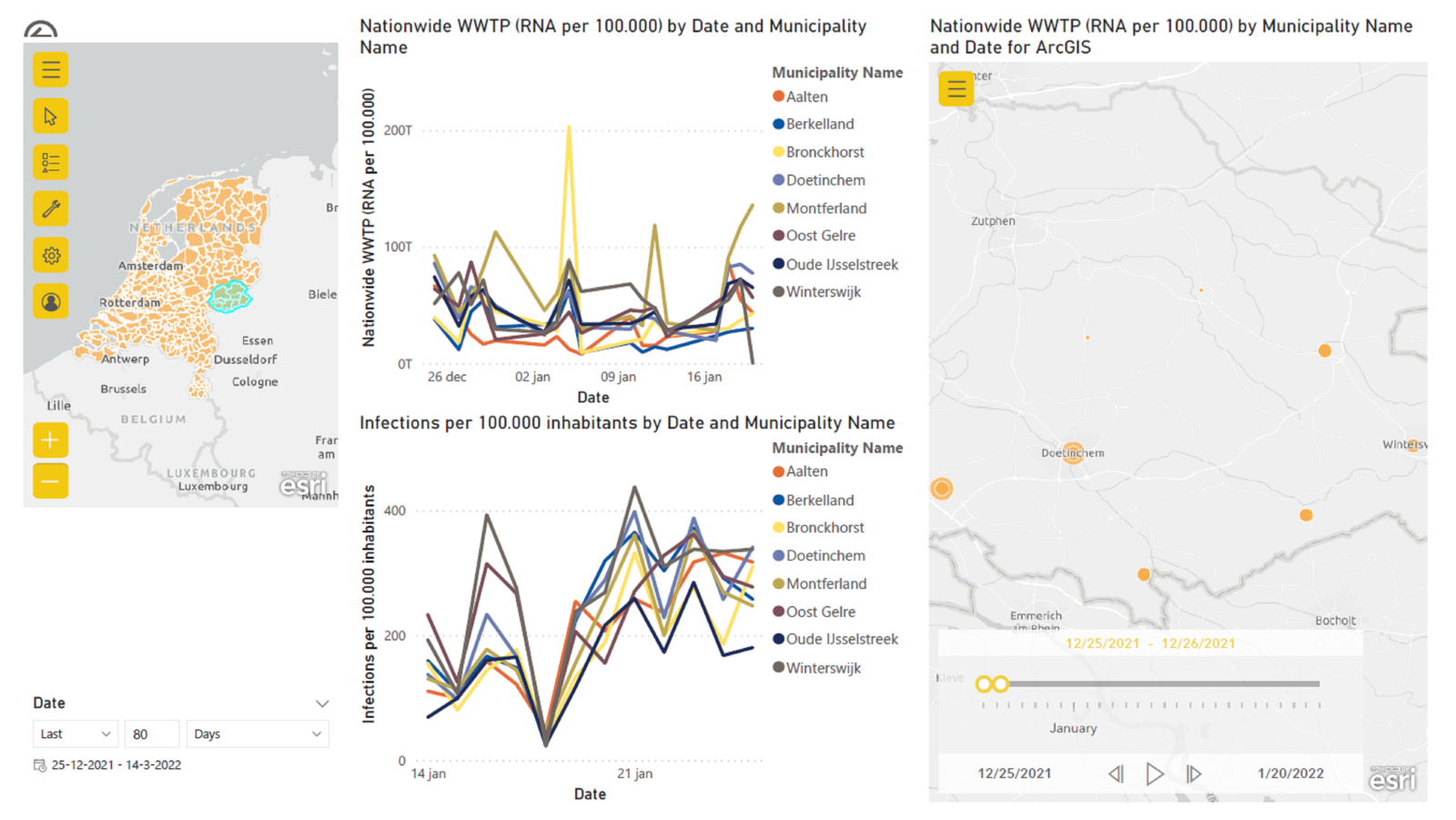
KPI correlation between parameters
On this page, the user can select a specific reference date. On this date all parameters (infections, hospitalisations and nationwide WWTP) are set to 100%. In this way, their correlation in time can be seen. A user can select a specific municipality or the entire country.
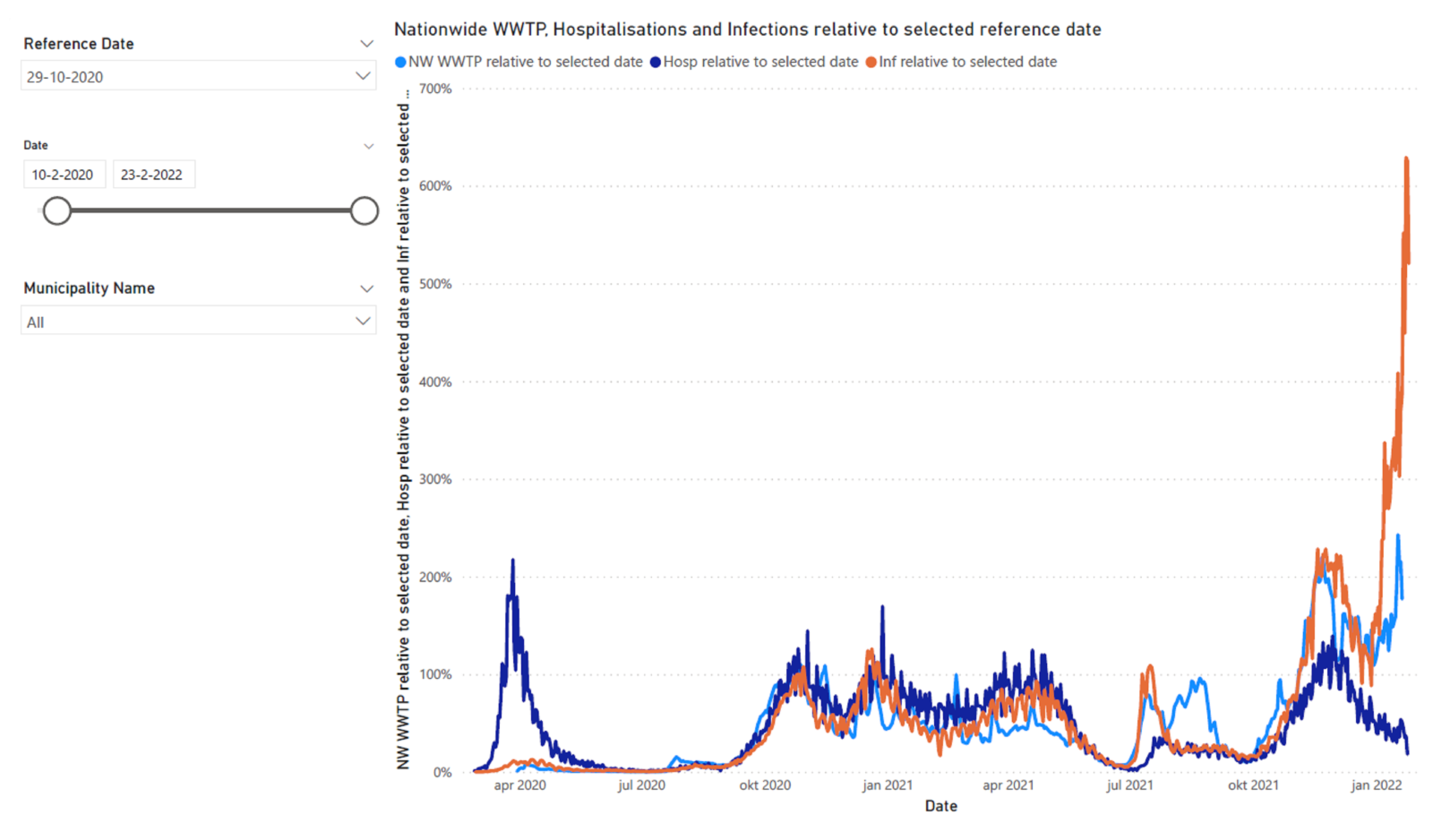
KPI Growth rate
This page shows the growth rate of hospitalisations and infections together with the absolute value of each parameter. Growth rate is defined as:
Where Number refers to either hospitalisations or infections.
Growth rate is an early warning tool. The user can select the municipality and the date range.
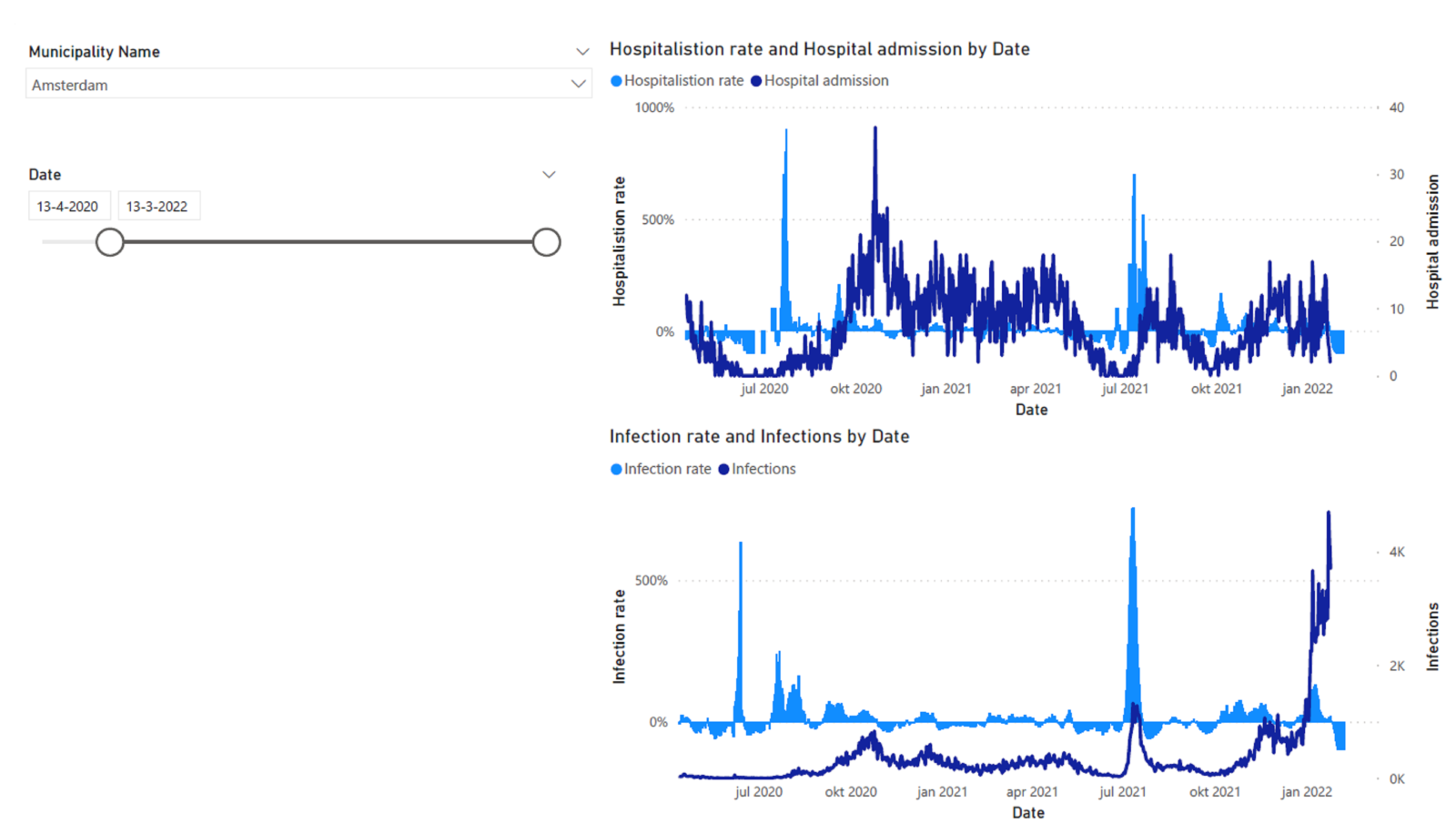
KWR detail
This page examines data quality of KWR WWTP measurements by comparison against flow, nationwide WWTP and by showing raw data in a table.
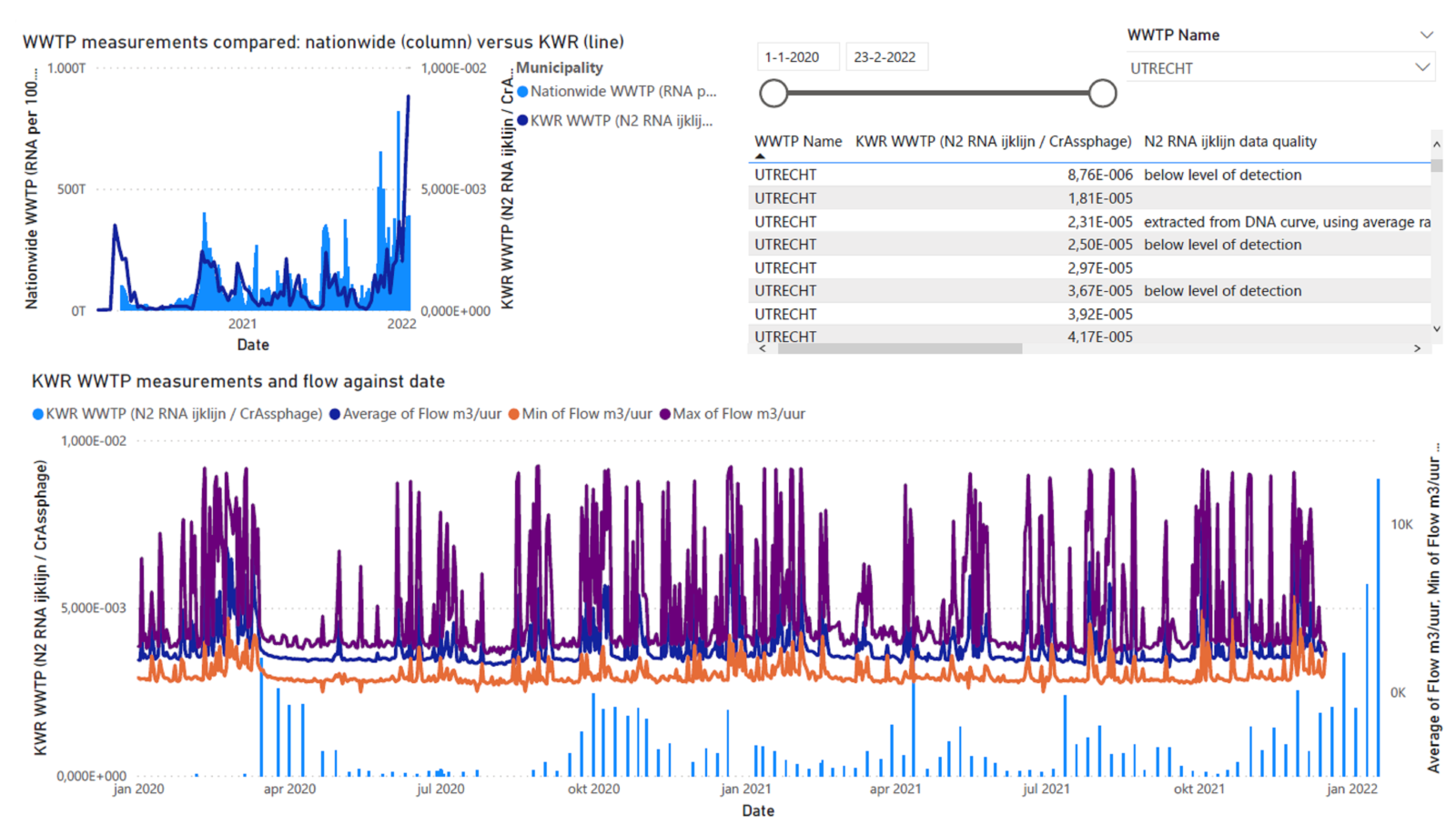
This dashboard is the first in the Netherlands that allows for direct comparison of parameters, uses maps, new KPIs and displays data quality information. It is recommended to combine this dashboard with other international initiatives such as the Johns Hopkins University & Medicine dashboard[1] or link it to W-Sphere[2], where KWR is already collaborating on.
South Africa data
For the South African data receive from Waterlab, the graphs were created in Excel.
The target genes specific for SARS-COV-2 are the E gene, RdRP gene, N gene:
-
Sarbecovirus (E gene)
-
SARS-CoV-2 (N gene)
-
SARS-CoV-2 (RdRP gene)
If any of the three resulted in a positive, then the test was indicated as positive for the presence of SARS-COV-2.
In the figure below, the consolidated, scaled Ct values for the three SARS-COV-2 target genes, as tested in South Africa, are summarised for each sampling site per week.
Ct values are converted into “scaled” values as shown in the legend, where a higher Ct value correlates to a lower presence of SARS-COV-2 in the samples measured, making graphs and data more difficult to compare as the expectation for the values is inverted. This is also highlighted by the increased intensity of the colours chosen (from green to purple), a so-called warning system. The values are in a +2 +4 +6 +8 and +10 range which is reflective of how other results are being expressed within South Africa e.g. by the MRC and NICD, who show results as genome copies per millilitre in the log range[3]. Some of the results are close to 6 logs per ml, i.e. the scale ranges from 0 to 6. The data from the Ct values could also be split differently, however whatever is chosen is arbitrary, as long as it can be easily understood.
The results have been categorised using the scale below.
A time series slider of the Ct values scaled, can be found under the tab "Interactive Mapping".
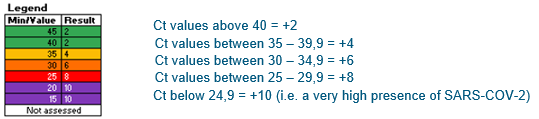
Blank cells in this data set indicate weeks in which “no assessments” or sampling was performed. The “Assessment %” in the table indicates the percentage of sampling done (or measurement from the sample) in total, as per the split weeks, i.e. column 1 shows that 60% of the weeks had at least 1 sample/measurement, while 40% remained unassessed.
In South Africa, retrieving an overview of this information for specific provinces (or the country) is difficult due to the lack of infrastructure throughout the areas targeted. In this study, two different types of sampling sites were included, namely untreated sewage at wastewater treatment works (as in the Netherlands) as well as in other waterbodies, i.e. rivers, streams and runoff in or near informal settlements, to represent the 40% of South Africans who do not have access to waterborne sanitation. To standardise information across these sites, additional information would be required, such as water ingress, flow rate and area rainfall. These additional data sets were not included in the scope of this project, but are important considerations for future projects.
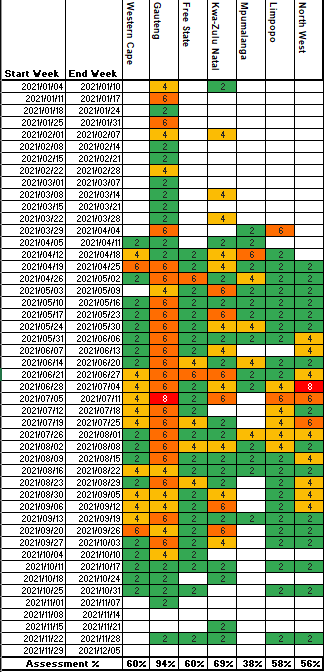
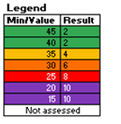
As mentioned previously CrAssphage is a relevant marker for faecal contamination in water. This means that if we pick up CrAssphage, but no SARS-COV-2 genes, we can derive that human faeces was present, but no COVID-19 in the community. For clarity, COVID-19 is the illness caused by the SARS-COV-2 virus. Additionally, we show that the methods can be used to detect more than SARS-COV-2. The Norovirus and Hepatitis E virus, for example, can be detected as people become ill in a community. They may result in similar symptoms to COVID-19, but are not epidemic or pandemic related e.g. an 'outbreak' of norovirus can occur from contaminated food at an event / restaurant etc. These additional viruses were measured by Waterlab as well.
Below the comparative table of SARS-COV-2, CrAssphage, Norovirus and HEV for Gauteng only.
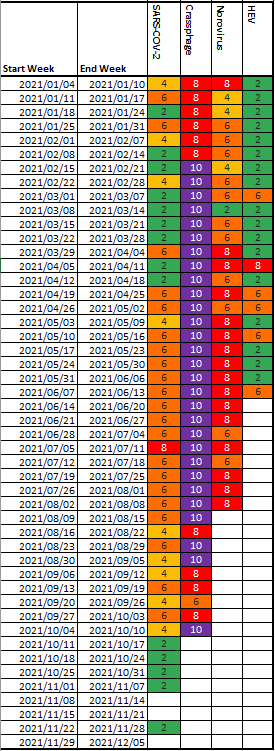
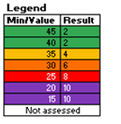
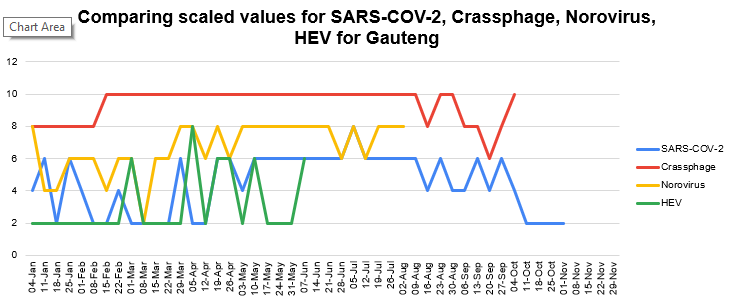
From the figures above, a high presence of faecal contamination is apparent throughout the research period (CrAssphage). SARS-CoV-2 is present throughout, however becomes more consistent between May and October of 2021, while the Norovirus and Hepatitis E virus fluctuate throughout the analysis period (measured only until August and May respectively).
SARS-CoV-2 comparison: The Netherlands and South Africa
Below we compare the target genes (in Ct values) for SARS-CoV-2 between the Netherlands (KWR data) and South Africa (Waterlab data) using the same scale as in Section 4.3.2.
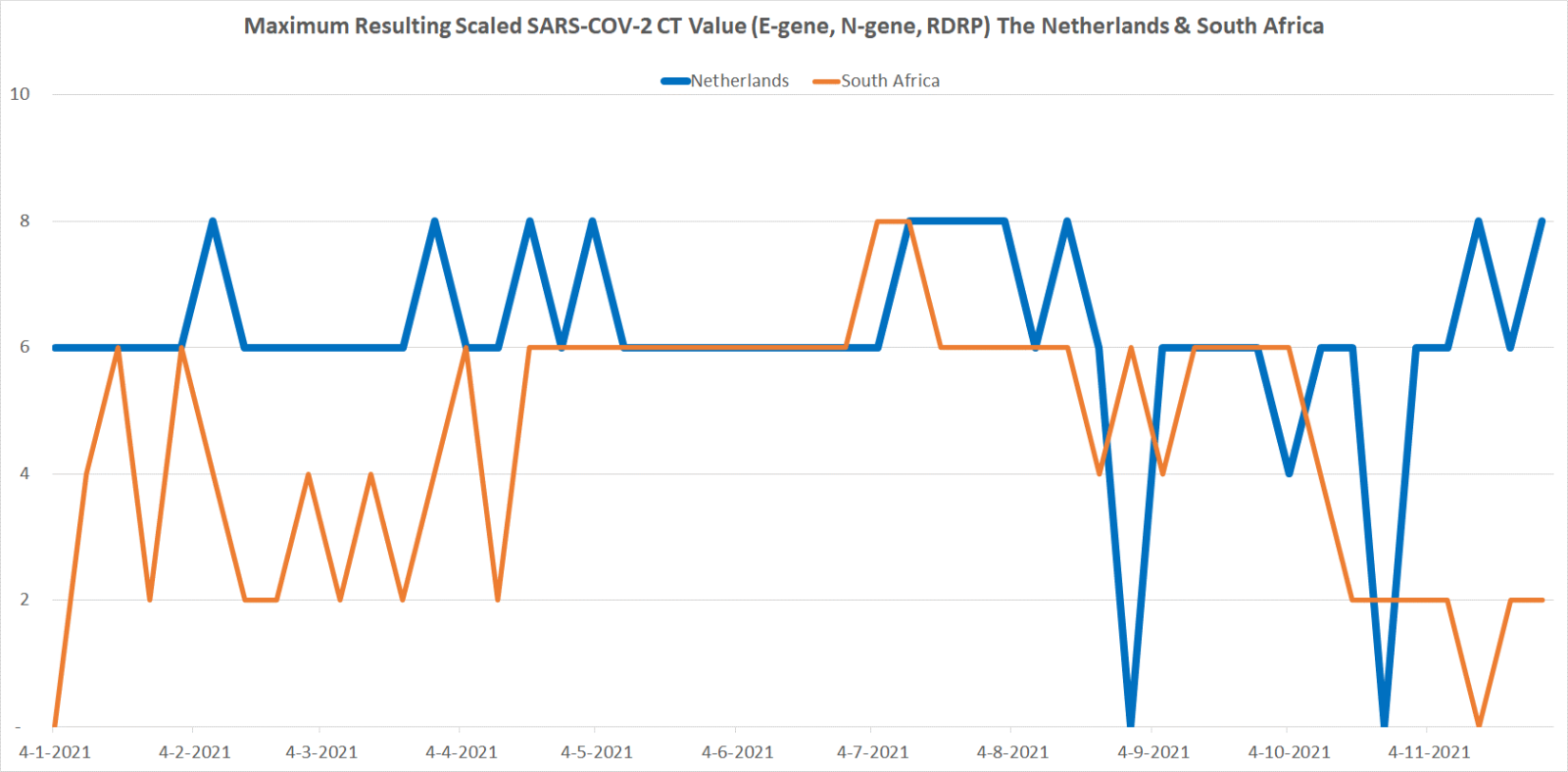
The figure above shows the comparative scaled Ct values for South Africa vs the Netherlands. The graph uses the maximum scaled value for each week across all sites for South Africa and the Netherlands respectively. The methodology used here is as follows: initial sampling data provided in Ct values, the minimum of these Ct values (strongest concentration) is then allocated per site per week, the minimum of the resulting Ct values are taken, this time for the E-gene and N-gene Ct. This minimum is converted using the scale listed in 4.3.2 above.
Some data points in South Africa are measured in rivers, while the rest were measured at wastewater treatment works. Without flow/concentration information these numbers cannot be properly compared against each other. A further point to note is that in this graph we have used the MAX scaled value (MIN Ct) always, but depending on the expectation one could also use average values. The MIN scaled value is not advisable though, as this would be a view of the low end of these results.
In general, the methodology used by KWR recovers more RNA, so their numbers or categorical data will always be higher for their data (Dutch) than for the Waterlab (South African) data, as can been in the figure above. As long as we are able to see changes for a new wave or a declining wave then such graphs serve the purpose of monitoring, and eventually predicting, the spread of the virus.
From this we can conclude that using categorised Ct values is a simple and reliable method to allow the comparison of data from different laboratories, making use of different extraction and identification procedures for the viral RNA, across geographical areas. This will enable trends of increasing or decreasing COVID-19 incidence to be observed over time.
- 1 https://coronavirus.jhu.edu/map.html
- 2 https://www.waterpathogens.org/about-w-sphere
- 3 https://www.samrc.ac.za/wbe/How-To-Guide.pdf